|
|
● LoveCS(Date:2022.5.13).
More
J. Wang, A. Ma, Y. Zhong, Z. Zheng, L. Zhang. “Cross-sensor domain adaptation for high spatial resolution urban land-cover mapping: From airborne to spaceborne imagery”,
Remote Sensing of Environment, vol 277, pp. 113058, 2022, doi: https://doi.org/10.1016/j.rse.2022.113058.
|
|
● Auto-AD(Date:2021.12.21).
Python Code
S. Wang, X. Wang, L. Zhang and Y. Zhong, "Auto-AD: Autonomous Hyperspectral Anomaly Detection Network Based on Fully Convolutional Autoencoder," in
IEEE Transactions on Geoscience and Remote Sensing, vol. 60, pp. 1-14, 2022, Art no. 5503314, doi: 10.1109/TGRS.2021.3057721.
|
|
● LoveDA(Date:2021.10.21).
More
J. Wang, Z. Zheng, A. Ma, X. Lu, Y. Zhong. “LoveDA: A Remote Sensing Land-Cover Dataset for Domain Adaptive Semantic Segmentation”, in
Proceedings of the Neural Information Processing Systems Track on Datasets and Benchmarks (NeurIPS), vol 1, 2021.1.
|
|
● ChangeStar(Date:2021.8.31).
More
Z. Zheng, A. Ma, L. Zhang, Y. Zhong. “Change is Everywhere: Single-Temporal Supervised Object Change Detection in Remote Sensing Imagery”, in
IEEE/CVF International Conference on Computer Vision (ICCV), 2021.
|
|
● FactSeg(Date:2021.7.18).
More
A. Ma, J. Wang, Y. Zhong, Z. Zheng. “FactSeg: Foreground Activation Driven Small Object Semantic Segmentation in Large-Scale Remote Sensing Imagery”, in
IEEE Transactions on Geoscience and Remote Sensing, doi: 10.1109/TGRS.2021.3097148 (Accepted). |
|
● SAED(Date:2021.6.26).
MATLAB code
X. Wang, Y. Zhong, C. Cui, L. Zhang and Y. Xu, "Autonomous Endmember Detection via an Abundance Anomaly Guided Saliency Prior for Hyperspectral Imagery," in
IEEE Transactions on Geoscience and Remote Sensing, vol. 59, no. 3, pp. 2336-2351, March 2021, doi: 10.1109/TGRS.2020.3001353.
|
|
● FPGA (Date:2020.5.28).
Python code
Z. Zheng, Y. Zhong, A. Ma, and L. Zhang, "FPGA: Fast Patch-Free Global Learning Framework for Fully End-to-End Hyperspectral Image Classification,"
IEEE Transactions on Geoscience and Remote Sensing, vol. 58, no. 8, pp. 5612-5626, Augest. 2020.
|
|
●
AFCMOMA (Date: 2016.4.21, V2.0).
code and data
A. Ma, Y. Zhong, and L. Zhang, "Adaptive Multiobjective Memetic Fuzzy Clustering Algorithm for Remote Sensing Imagery,"
IEEE Transactions on Geoscience and Remote Sensing, vol. 53, no. 8, pp. 4202-4217, 2015.
|
|
● SGSNMF(Date:2018.5.4).
MATLAB code
X. Wang, Y. Zhong, L. Zhang and Y. Xu, "Spatial Group Sparsity Regularized Nonnegative Matrix Factorization for Hyperspectral unmixing,"
IEEE Transactions on Geoscience and Remote Sensing, vol. 55, no. 11, pp. 6287-6304, 2017.
|
|
● SGIDN
Python code
Zhong, Y., W. Li, X. Wang, S. Jin and L. Zhang. "Satellite-ground integrated destriping network: A new perspective for EO-1 Hyperion and Chinese hyperspectral satellite datasets."
Remote Sensing of Environment237: 111416.
|
|
● SSBFC
Python code
Zhao B , Zhong Y , Zhang L . A spectral–structural bag-of-features scene classifier for very high spatial resolution remote sensing imagery[J].
Isprs Journal of Photogrammetry & Remote Sensing, 2016, 116(Jun.):73-85.
|
|
● DMTM
Python code
Zhao B , Zhong Y , Xia G S , et al. Dirichlet-derived multiple topic scene classification model fusing heterogeneous features for high resolution remote sensing imagery[J].
IEEE Transactions on Geoence and Remote Sensing, 2015, 54(4).
|
|
|
|
|
●
LoveDA dataset (Date:2022.1.13).
download
1.5987 high spatial resolution (0.3 m) remote sensing images from Nanjing, Changzhou, and Wuhan
2.Focus on different geographical environments between Urban and Rural
3.Advance both semantic segmentation and domain adaptation tasks
4.Three considerable challenges:
- Multi-scale objects
- Complex background samples
- Inconsistent class distributions.
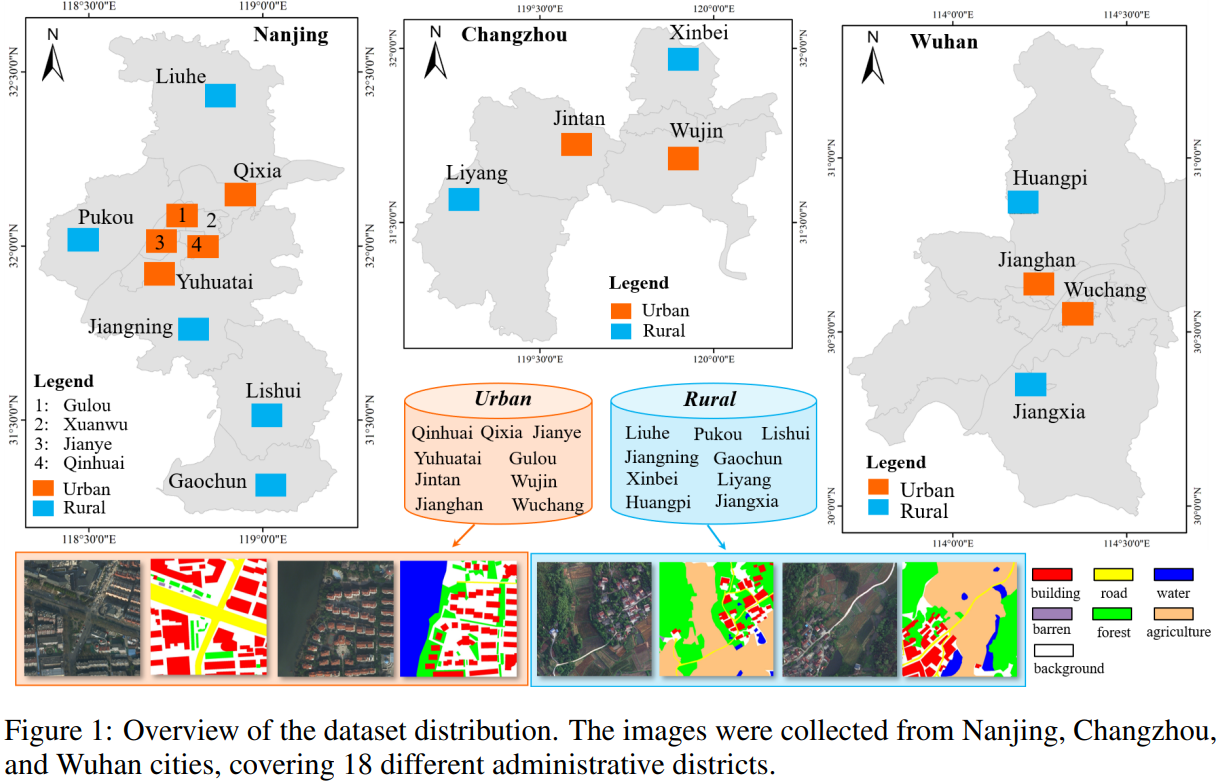 |
Fig. 1.LoveDA dataset
|
|
|
●
WHU-Hi dataset for target detection (Date: 2021.12.21)
download |
|
●
The Large-Scale Road Validation Dataset (Date: 2021.03.29)
download
The large-scale road validation (LSRV) dataset was built for the validation of the road detection task, which contains the images from Boston and its surrounding cities in the United States, Birmingham in the United Kingdom and Shanghai in China.
image |
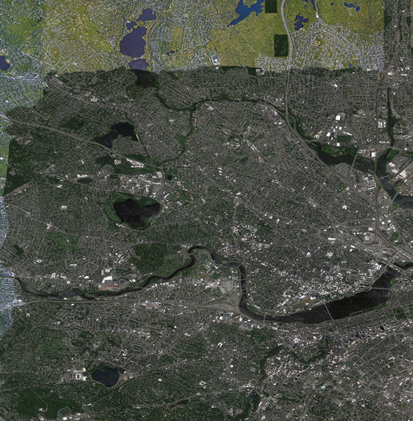 |
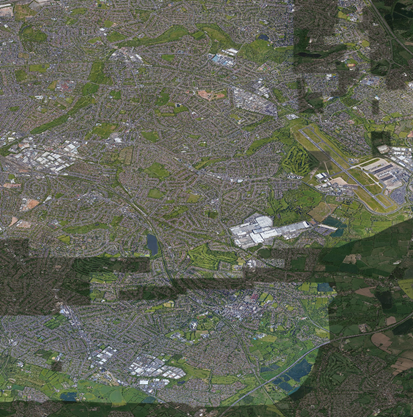 |
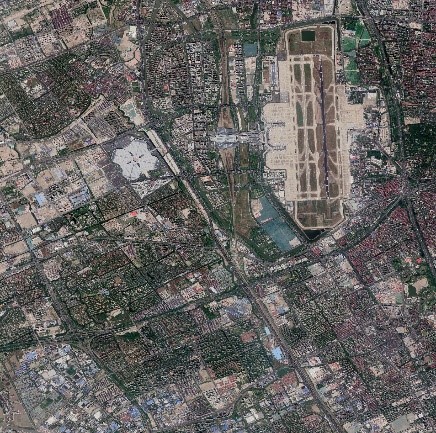 |
mask |
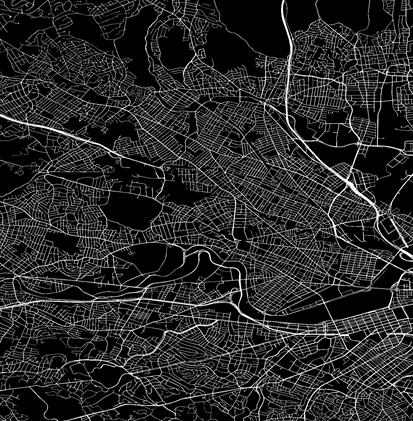 |
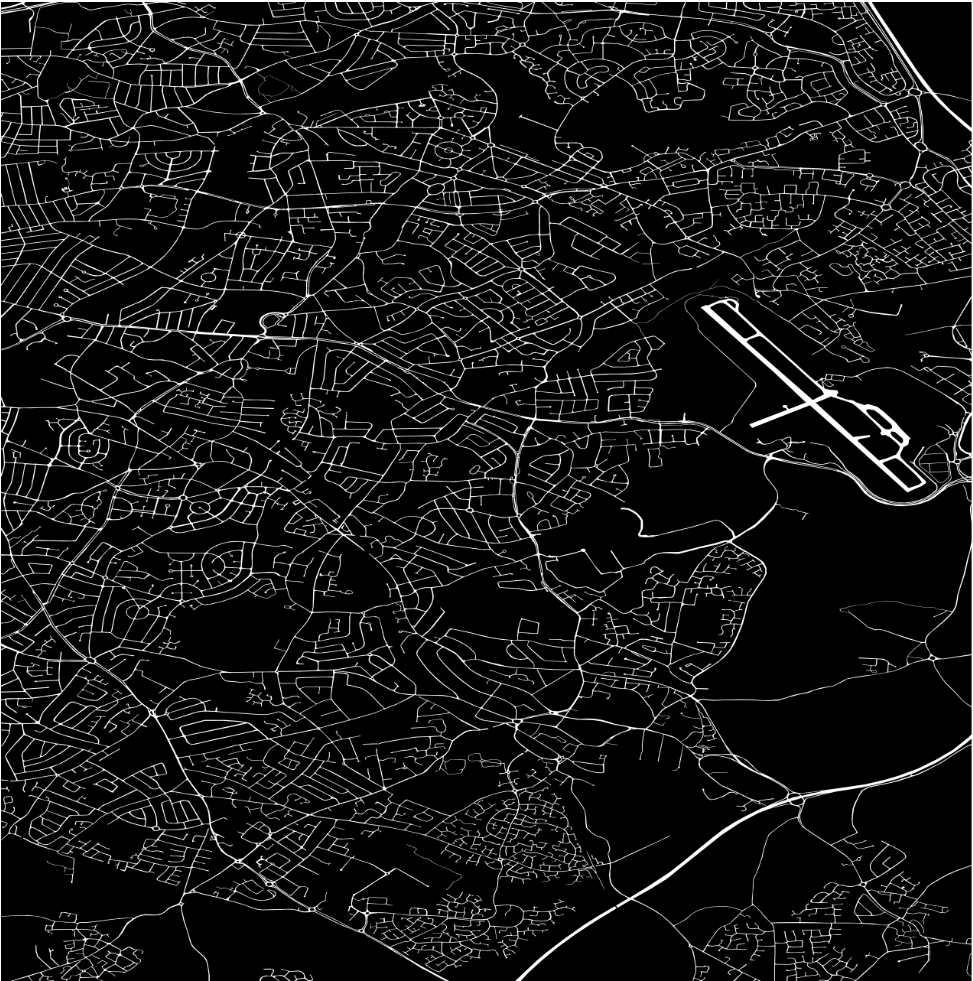 |
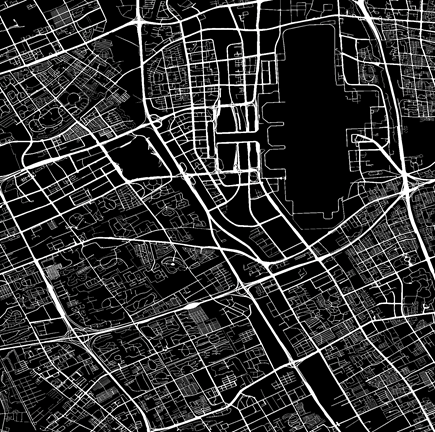 |
1 |
Boston and its surroundings, in the U.S |
Birmingham, in the UK |
Shanghai, in China |
|
Fig. 1.The large-scale road validation dataset.
|
|
|
●
WHU-Hi dataset (Date:2020.10.08).
download
The Wuhan UAV-borne hyperspectral image (WHU-Hi) dataset was built for hyperspectral image classification and precise crops identification, which contains three individual UAV-borne hyperspectral datasets: WHU-Hi-LongKou, WHU-Hi-HanChuan, and WHU-Hi-HongHu. All the datasets were acquired in farming areas with various crop types in Hubei province, China, via a Headwall Nano-Hyperspec sensor mounted on a UAV platform. In addition, the WHU-Hi dataset could serve as a benchmark dataset for hyperspectral image classification studies.
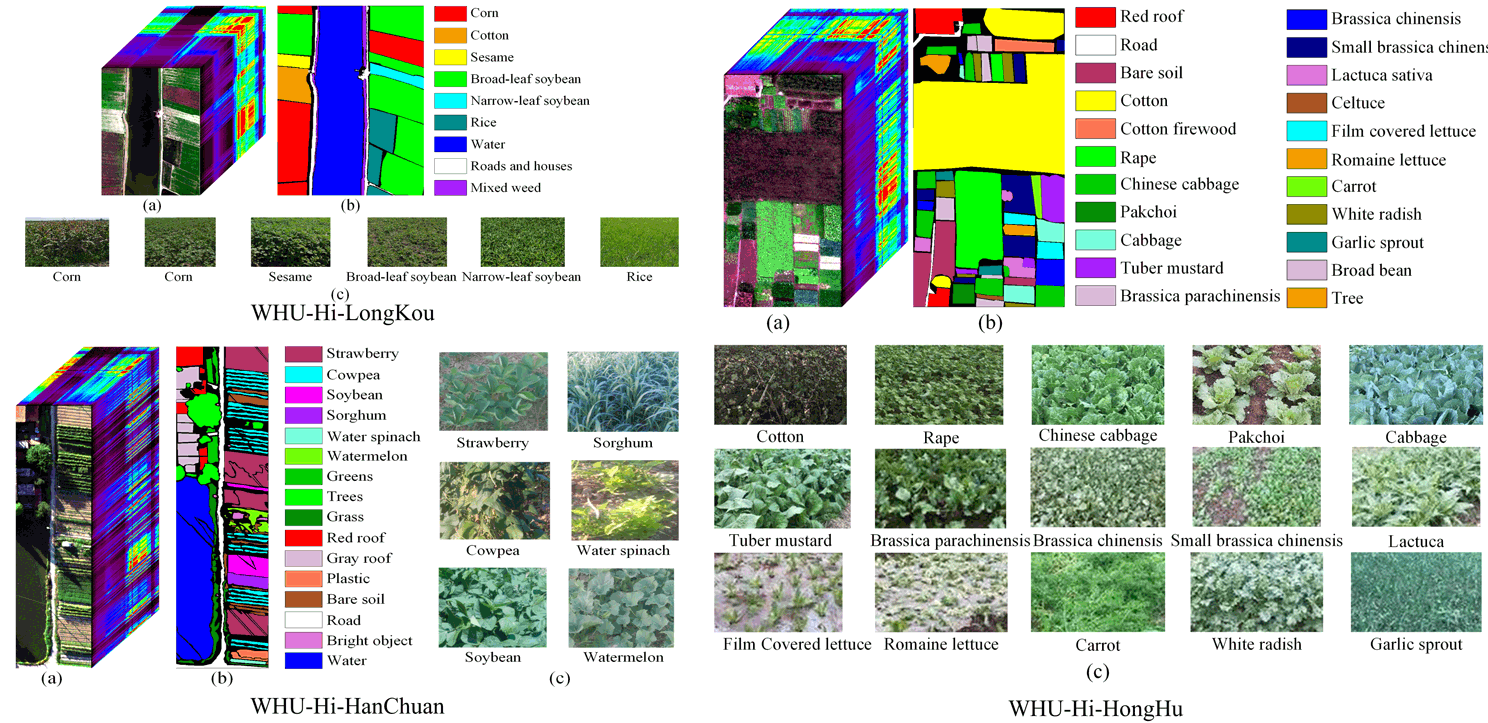 |
Fig. 1.WHU-Hi dataset
|
|
|
● The Google image dataset of SIRI-WHU (Date:2016.4.19).
download
This is a 12-class Google image dataset of SIRI-WHU meant for research purposes.
There are 200 images for each of the following classes:
Agriculture |
Commercial |
Harbor |
Idle land |
Industrial |
Meadow |
Overpass |
Park |
Pond |
Residential |
River |
Water |
Each image measures 200*200 pixels, with a 2-m spatial resolution.
This dataset was acquired from Google Earth (Google Inc.) and mainly covers urban areas in China, and the scene image dataset is designed by RS_IDEA Group in Wuhan University (SIRI-WHU).
Please cite the following paper when publishing results that use this dataset:
1. B. Zhao, Y. Zhong, G.-s. Xia, and L. Zhang, "Dirichlet-Derived Multiple Topic Scene Classification Model Fusing Heterogeneous Features for High Spatial Resolution Remote Sensing Imagery," IEEE Transactions on Geoscience and Remote Sensing, vol. 54, no. 4, pp. 2108-2123, Apr. 2016.
2. B. Zhao, Y. Zhong, L. Zhang, and B. Huang, "The Fisher Kernel Coding Framework for High Spatial Resolution Scene Classification," Remote Sensing, vol. 8, no. 2, p. 157, doi:10.3390/rs8020157 2016.
3. Q. Zhu, Y. Zhong, B. Zhao, G.-S. Xia, and L. Zhang, "Bag-of-Visual-Words Scene Classifier with Local and Global Features for High Spatial Resolution Remote Sensing Imagery," IEEE Geoscience and Remote Sensing Letters, DOI:10.1109/LGRS.2015.2513443 2016.
● The USGS image dataset of SIRI-WHU (Date: 2016.4.19).
This is a 4 class USGS image dataset of SIRI-WHU annotation image meant for research purposes.
The USGS image dataset of SIRI -WHU is a color aerial orthophotographs mainly contains the following 4 scene classes:
Farm |
Forest |
Residential |
Parking lot |
The large image measures 10000*9000 pixels, with a 2 ft spatial resolution.
This dataset was acquired from USGS, covering Montgomery Country, Ohio, USA, and the scene image dataset is designed by RS_IDEA Group in Wuhan University (SIRI-WHU).
Please cite the following paper when publishing results that use this dataset:
Y. Zhong, Q. Zhu, and L. Zhang, "Scene Classification Based on the MultiFeature Fusion Probabilistic Topic Model for High Spatial Resolution Remote Sensing Imagery," IEEE Transactions on Geoscience and Remote Sensing, vol. 53, no. 11, pp. 6207-6222, Nov. 2015.
Zhong Y, Cui M, Zhu Q, and L. Zhang. "Scene classification based on multifeature probabilistic latent semantic analysis for high spatial resolution remote sensing images," Journal of Applied Remote Sensing, vol. 9, no. 1, 2015. |
|
|