IS-RoadDet:
Road Vector Graph Detection with Intersections and Road Segments
from High Resolution Remote Sensing Imagery
TGRS 2024
- Ruoyu Yang
- Yanfei Zhong
- Yinhe Liu
- Dingyuan Chen
- Yang Pan
Abstract
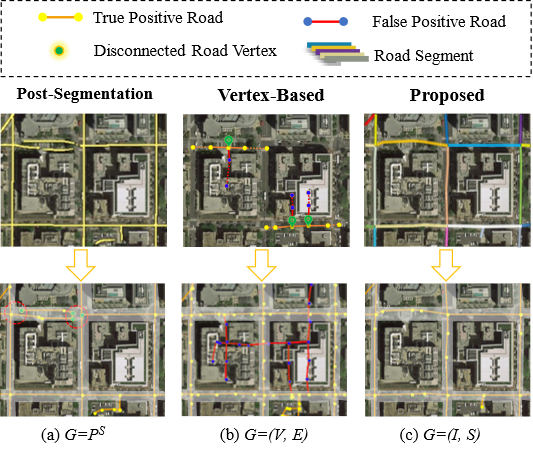
Extracting road vector graphs with high accuracy from high resolution remote sensing imagery presents a significant challenge. The prior end-to-end algorithms have typically modeled the road graph as a general graph structure with vertices and edges, denoted as G=(V,E), which is a standard approach in graph generation tasks. However, the traditional graph representation with vertices and edges, utilizing very small edge units, can conflict with the road network’s geometric structure and the inherent features of road instances, leadings to issues such as false positives and disconnected roads. In this paper, the IS-RoadDet framework is proposed to generate a road vector graph with Intersections (I) and road Segments (S), denoted as G=(I,S), which leverages the minimum road topology unit features of road to improve road topology. Compared to G=(V,E), instead of detecting a large number of vertices to maintain the road topology connectivity, uses road segments with minimum road units to avoid false positives. In IS-RoadDet, the Intersection and road Segment Detector (ISDetector) is introduced to detect intersections and road segments as independent instance objects with joint learning, and an Intersection Connection Strategy (ICS) is designed to establish connectivity between road segments with intersections. Empirical experiments conducted on the SpaceNet3 and Sat2Graph datasets substantiated the superior performance of the proposed road segment modeling method.
IS-RoadDet
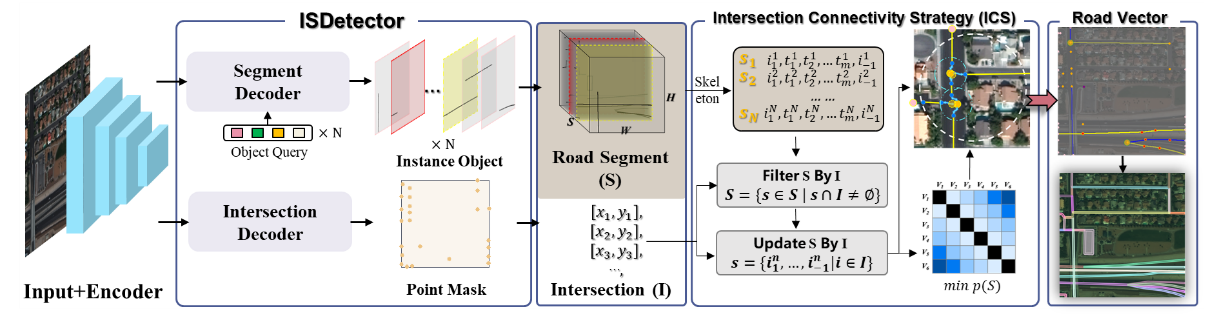
In this paper, we propose the IS-RoadDet framework, a method for road vector graph detection, which aims to address false positive and disconnected roads by the minimum road topology unit model, . Our main contributions can be summarized as follows: 1) The IS-RoadDet framework is proposed to generate a road vector graph with from VHR remote sensing imagery. The IS-RoadDet framework firstly represents the road topology based on the minimum road topology unit, effectively distinguishing road topological points from non-topological points in the different modeling units to balance the precision and connectivity of road vector graph generation. 2) The ISDetector is proposed to obtain the road segments (S) and intersections (I) of roads. It considers the spatial correlation of road segments and topological points and uses joint learning to optimize the road segment detection and intersection segmentation. 3) The Intersection Connectivity Strategy (ICS) is introduced to combine road segments and intersections into a road vector graph. Meanwhile, the ICS considers the minimum connectivity property of the non-topological vertices in the road segments to mitigate the occlusion problem.
Experiments
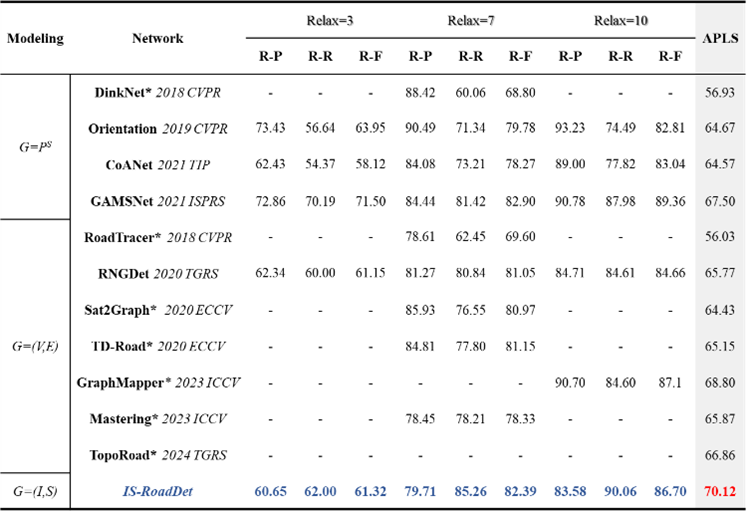
In order to verify the performance of the proposed IS-RoadDet framework, it was tested on the SpaceNet3 dataset and Sat2Graph dataset and compared with the previous post-segmentation based methods of DinkNet , Orientation , CoANet , and GAMSNet , and the previous vertex-based methods of RoadTracer , RNGDet , Sat2Graph , TD-Road , Mastering , TopoRoad GraphMapper and SAM-Road . To verify the validity of IS-RoadDet, SpaceNet dataset and Sat2Graph dataset was used to conduct experiments.
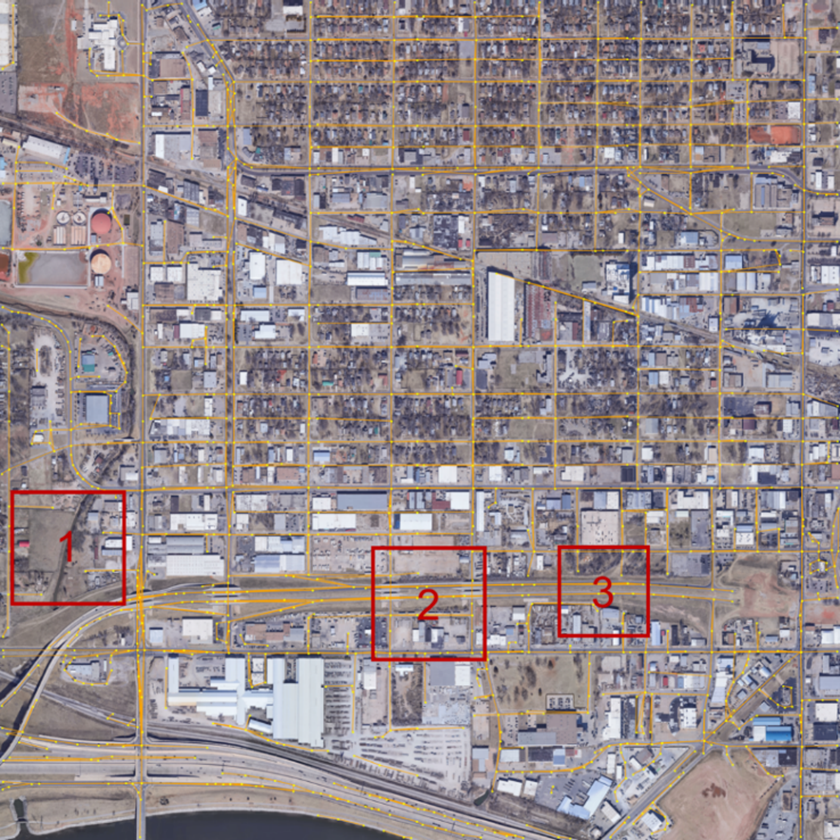
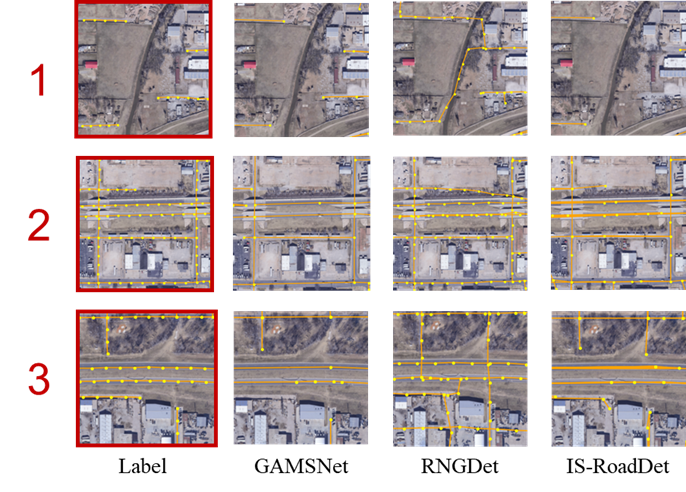
BibTeX
journal={IEEE Transactions on Geoscience and Remote Sensing},
title={IS-RoadDet: Road Vector Graph Detection with Intersections and Road Segments from High Resolution Remote Sensing Imagery},
year={2024},
doi={10.1109/TGRS.2024.3483113}
}