Motivation
Advanced interpretation of hyperspectral remote sensing images benefits many precise Earth observation tasks. Recently, visual foundation models have promoted the remote sensing interpretation but concentrating on RGB and multispectral images. Due to the varied hyperspectral channels, existing foundation models cannot process new sensor types directly and face image-by-image tuning situation, imposing great pressure on hardware and time resources.
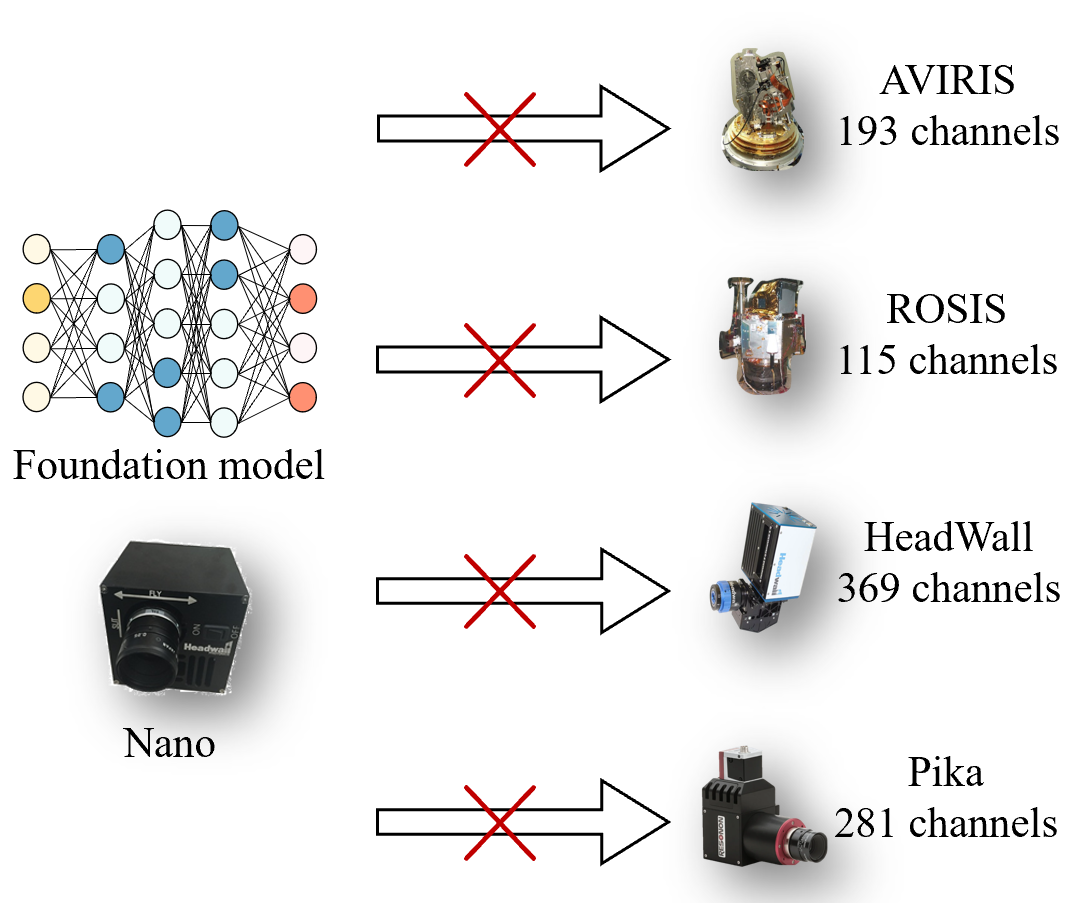